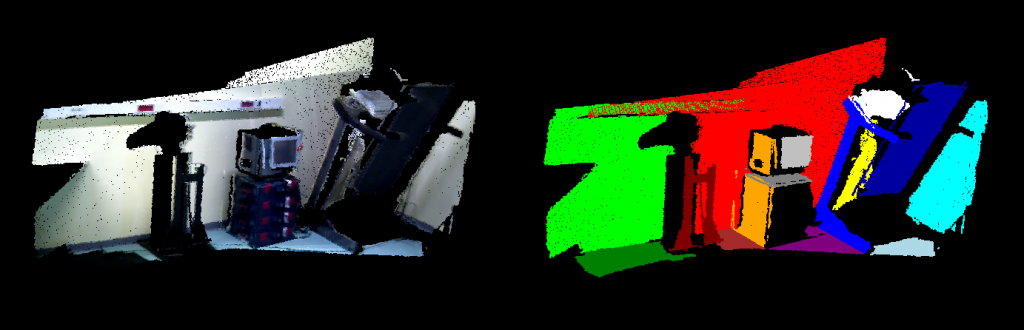
Date: 07.2019 – 07.2021
Budget: 139960 PLN
Project Manager: Jan Wietrzykowski
Funder: NCN
The project, funded by NCN under grant no. UMO-2018/31/N/ST6/00941, concerns geometric description of indoor scenes in the context of localization of an autonomous agent. The aim is to find new methods for scene description using geometric features. These methods should support the task of finding the pose of an agent with respect to a known map of the environment. The need for an efficient and practical way to describe indoor scenes in man-made environments became evident during our previous research dealing with global localization. This challenging task involves finding the agent pose without any prior knowledge about its whereabouts by comparing the local descriptions of the scene. The difficulties that arose when developing the global localization system prompted us to investigate entirely new ways to decompose a scene perceived by passive stereo cameras into high-level geometric features, such as planar and line segments.
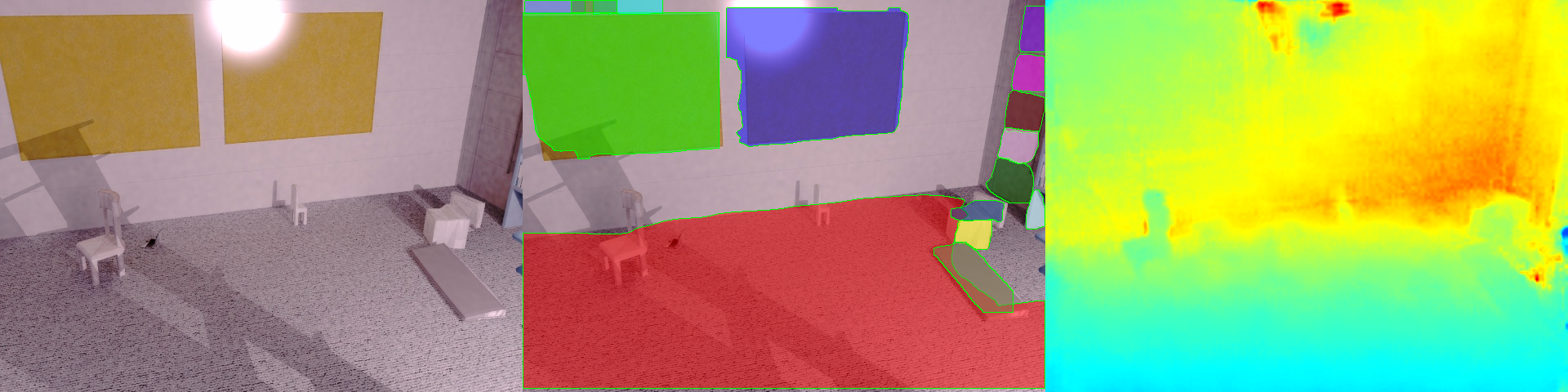
Taking into account the recent trends in computer vision and pattern recognition and turning towards data-driven paradigm, we choose the Deep Neural Networks (DNN) as a base for our research. While many methods for semantic segmentation and feature extraction employing some form of deep learning have been proposed recently, few of them concern high-level geometric features.
This project has two main scientific goals. The first one is to develop new methods for data-driven, robust scene decomposition using a minimum amount of supervision in learning to enable operation in real-world scenarios. The second goal is to investigate how to employ evaluation of the localization accuracy in the module that learns the feature matching function for high-level geometric features.
Evaluation of the proposed methods will be done using real-world data gathered in indoor, office-like environments. One of the datasets for this purpose was created during the SMILE project in the TERRINet framework.